AI.models could predict outcomes in trauma
根据美国外科医学院临床大会2020年的两项研究研究,AI在医疗保健环境中提高了改善救护车运输的交通事故受害者的生存和结果,提前取得了早期成功。
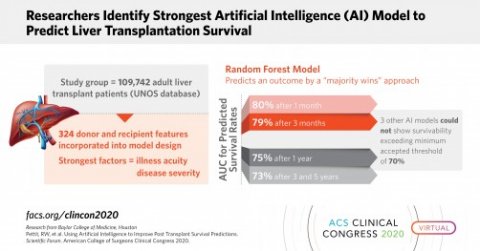
两项研究评估了如何人工智能can crunch massive amounts of data to support decision-making by surgeons and other care providers at the point of care.
在一项研究中,明尼苏达大学的研究人员应用了先前发表的AI方法自然语言处理(NLP)1分类治疗需求和医疗干预措施为22,529台机动车崩溃患者,紧急医疗服务(EMS)人员运送到ACS验证的水平创伤明尼苏达州的中心。根据2016年的学术学院,工程和医学,20%的医疗伤害死亡可能预防,代表研究人员寻求解决的质量差距。
Reviewing the performance of EMS teams to profile potentially preventable deaths can enable quality improvement efforts to reduce these deaths. “Currently this process for performance review is manual, time-consuming, and expensive,” said senior author Christopher James Tignanelli, MD, FACS. “AI allows possible automation of this process.” NLP is an AI protocol that extracts key data from spoken or written text that providers—EMS personnel in this study—enter into the electronic record as a key component of their report. Dr. Tignanelli is an assistant professor ofsurgery,在明尼苏达大学医学院,明尼苏达大学医学院医学院的急性护理手术分工。
在这项研究中,两个创伤外科医生独立并手动审查了1%的患者记录和确定的治疗需求和医疗干预的随机选择。为了评估AI系统的准确性,将手动测定与NLP测定进行比较。“总的来说算法Tignanelli博士说,以非常高的准确性执行。
通常在EMS人员进入他们的笔记to the electronic health record, oversight personnel cull through them and determine whether the patient received appropriate care, usually a week or so afterward. “That’s quite a labor-intensive process,” said presenting author Jacob Swann, MD, a burn and trauma fellow at Regions Hospital in St. Paul, Minn. “The goal of this project and what it validated was to automate a lot of those notes.”
NLP方法通过算法运行这些说明,将结果与后果性较少的结果分开。“这可以简化手动审查过程,”斯旺博士说。“它不是在准确级别进行的,允许您将医生脱离它,并说AI如果给予的标准或没有,则可以通过完全准确地确定,但它确实表现良好。”
The AI pipeline Dr. Swann and colleagues studied determined that only about one quarter (242 of 936) patients who needed an airway intervention actually got one before they arrived at the hospital, and that about two-thirds (110 of 170) of those who did not have adequate intravenous access and needed access into the bone, known as intraosseous (IO) access, during advance cardiac life support did receive IO access. “Being able to identify systemic errors allows you to improve the entire health system,” Dr. Swann said. “Having the ability to look at large aggregate data and go through 330,000 charts over several minutes with an AI-reading algorithm, to identify specific areas for potential improvement—whether it’s getting intravenous access in our patients or having problems with splinting long bone fractures—allows you separate the signal from the noise and then figure out where the problem lies.”
The “holy grail,” noted Dr. Swann, is to have an AI system that can listen and observe EMS personnel during en route care and assist with complex decision making by recommending care options in real time.
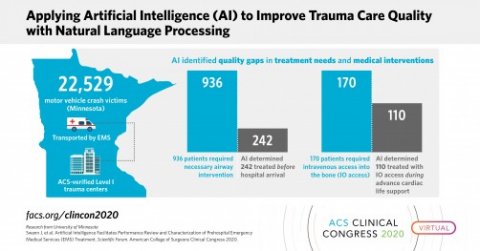
对于第二次AI研究,休斯顿贝勒医学院的研究人员测试了四种不同的机器学习模型,用于预测肝脏后生存transplantation. The two models that showed high accuracy for predicting survival are known as the Random Forest and AdaBoost models. Lead author Rowland Pettit, MD-PhD candidate at Baylor, explained that Random Forest (RF) is an ensemble learning method that combines the outputs of multiple decision trees and predicts an outcome by a “majority wins” approach.
The models took into account a total of 324 disease characteristics to determine survivability. The strongest factors were acuity of illness and the recipient’s disease course, Mr. Pettit said.
The study selected all 109,742 adult patients who had one liver transplant from the United Network of Organ Sharing database since its inception in 1984. The RF model showed an accuracy, reported as area under the curve, of 80 percent for predicting survival at one month, 79 percent at three months, 75 percent at one year, and 73 percent at three and five years. None of the other models showed predictability exceeding 70 percent. “The most readily accessible application of these models would be for regulation, providing immediate feedback to clinicians about their outcomes for the past year and how they and their centers performed compared to others,” Mr. Pettit said. “Being able to accurately predict whether a patient should have survived or not is crucial to then accurately providing feedback.”
他补充说,这种类型的AI模型也有可能与电子医疗系统和医生工作流集成,以提供基准。“在实时运行肝脏移植等候名单上的每只患者的综合模型都非常容易,并确定每个患者在一个,三年或五年内达到的概率,”他说。“这一步骤不是为临床医生做出决定,而是添加另一个临床医生辅助决策工具,为他们提供有关器官分配决策的定量数据。”
Source:美国外科医院